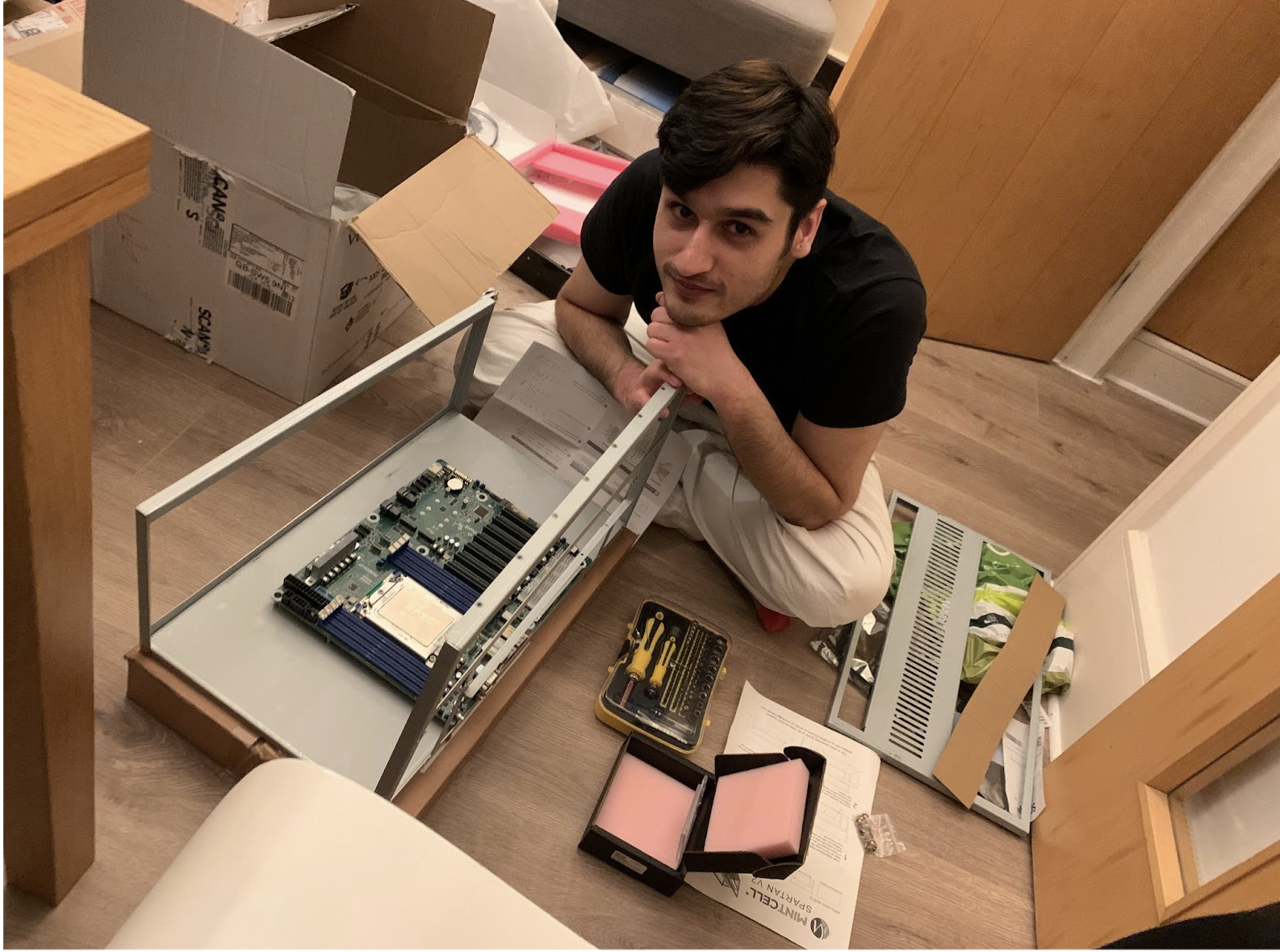
Introducing The Next Generation
Samyakh Tukra
Founder & CEO at Third Eye Intelligence LTD
Have you ever thought about what will happen to our bodies by the time we enter our early 70s? The future tends to fill us with fear, and since it’s not here now, it’s an easy thought to push aside.
Over time, the body deteriorates, weakening to a point where it cannot fight against the medical threats that haunt us. I witnessed it happening not too long ago, when my grandfather was admitted to an Intensive Care Unit (ICU) for a heart attack. Despite being in the highest echelon of care, where he was monitored 24/7, he died within five days due to multi-organ failure. A feeling of helplessness sank in. My family and I had forfeited my grandfather’s fate to the doctors in the ICU, and so had other families with their loved ones.
Suddenly, it hit me. This wasn’t just about my grandfather. There are more than 85 million patients admitted to the ICU annually for potential organ failure, yet only up to 50% of them survive.
I decided to shadow multiple doctors in the ICU and conducted more than 40 interviews. I found the underlying problem is that organ failure is often detected too late. Healthcare staff have to make life-or-death decisions in high-pressure settings while retaining and analysing mounds of information at speed. Hence, interventions are delayed and chances of survival for patients are low. This results in increased treatment costs as well as patient morbidity and mortality. Additional treatment costs are estimated at $220 billion globally, and 7 million lives could potentially be saved every year. With acute kidney injury alone, for instance, early detection and treatment could prevent 42,000 deaths in the UK annually, according to National Institute for Health and Care Excellence estimates.
The problem isn’t that clinicians don’t have suitable ways to intervene, but that they can’t plan for when to do so.
Current early warning systems (e.g. NEWS, Apache III) are static and tend to compute scores based on a pre-set combination of vitals to get a momentary risk-score for patient deterioration, giving few insights that would allow doctors to catch future deterioration in time. These systems fail to use the entirety of available digital patient data, only address specific organ-failure conditions, and are often not time-specific in their predictions. Hence, they lack the crucial ability to analyse and predict deterioration for more complex multi-organ conditions and have only limited usefulness. Current methods also fail to utilise the electronic health records (EHRs) comprising the patient’s entire digital footprint in real time. Mostly, this is because they can’t scale to that level of complexity or predictive power, but with the advent of machine learning this is no longer a limitation. With deep learning in particular, we can train a model to do such inferences.
To address this problem and give patients a fighting chance, I created Third Eye – a universal early warning system for organ failure based on an evolving AI algorithm. The AI processes and amalgamates Currently, this system can predict organ failure 36 hours before disease onset with 96% accuracy, so that clinicians have a longer time window to administer life-saving interventions before it’s too late.
The underlying technology is based around automated machine learning (AutoML). AutoML is a branch of AI where instead of humans designing AI models, we train another AI system to design better AI models. Third Eye’s system comprises two AI models, where one AI model – called designer – iteratively designs and optimises the other – candidate model – to attain peak prediction performance. The final candidate model is vastly superior to industry standard, hand-designed AI models, which are sequential and limited in nature. Our approach overcomes the limitations found in existing hand-designed AI models, such as lack of optimal AI design, lack of generalisability, inability to learn features from multiple input sources and more. Since our AI designs candidates from the inherent patterns of data itself, bias associated with human designs is significantly reduced.
We embed our AI in a support platform that provides actionable organ failure predictions to help ICUD staff make decisions. By integrating our software into current ICU workflows, we estimate an increase in survival chances for organ failure conditions by up to 65% – and up to 30% reduction in ICU costs.
Our goal is to bring this technology into the clinical environment very soon. We are about to enter a clinical trial to validate its performance in a real ICU, to ensure the system is stress-tested for all possible scenarios. There is a huge discrepancy between data used for validating machine learning models outside of the clinical setting and within, hence it’s vital as AI researchers we develop our systems robust to such cases. Over the next 20 months, we will be testing our system in the ICU while collating all the evidence required to achieve regulatory approval and get ready for market entry.
A look of our software:
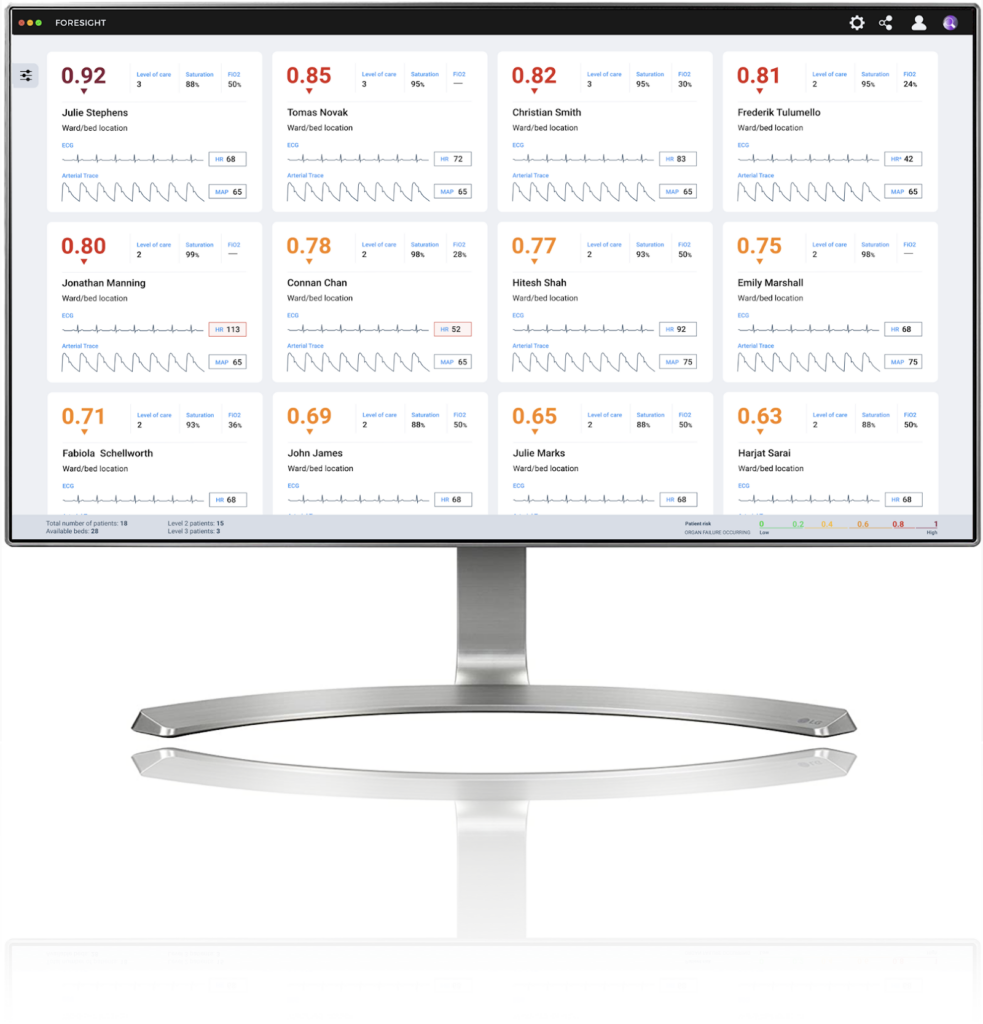